Tianwei Yin’s Adobe Research internship began with a plan to investigate 3D content generation. But by the end of the summer, he and his collaborators had developed a new one-step generative AI model that can create images 30 times faster than previous generative AI models, all with comparable image quality.
The work was the culmination of work by Yin, an MIT PhD student, alongside his internship mentor, then Adobe Research Scientist Michaël Gharbi, along with Adobe Research collaborators Research Scientist Richard Zhang, and Senior Principal Scientist Eli Shechtman, then Research Scientist Taesung Park, and MIT professors Frédo Durand and William T. Freeman.
The team is publishing its research, One-step Diffusion with Distribution Matching Distillation, at CVPR 2024, and they have continued collaborating together on the next version of the work.
In brainstorming sessions, Yin and the team sketched out a research plan that would involve working with diffusion models, a type of generative AI model. But they kept hitting the same stumbling block—training and working with the diffusion models would be too slow to get useful results in the span of a summer. So, they started thinking about the models themselves, and how to get them to generate images faster—which would decrease energy usage and cost.
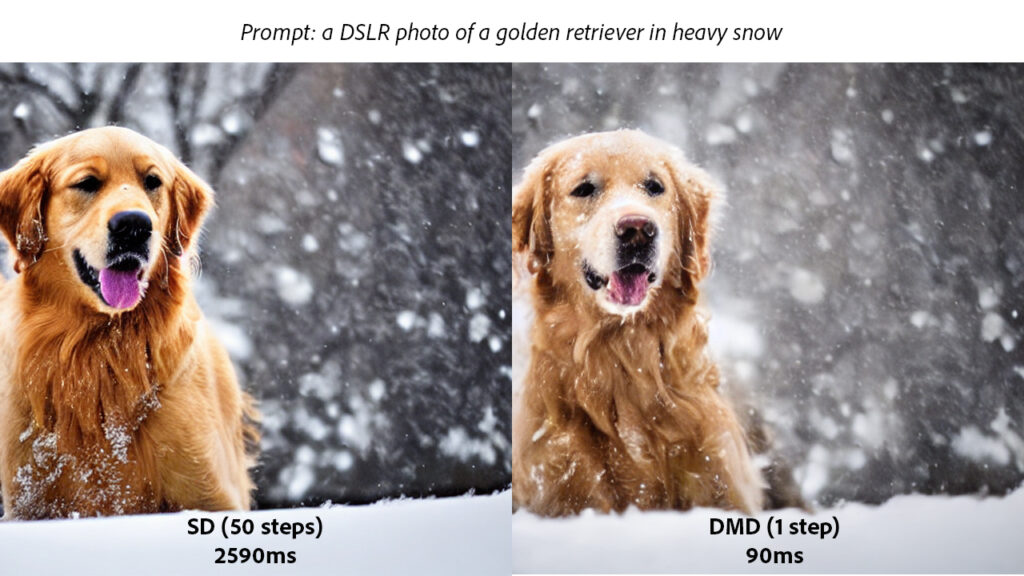
Going from as many as 1,000 steps to just a few
State-of-the-art generative AI models are slow and costly because the generation process is iterative and sequential. To generate a new image, the model begins with something that is more “noise” than image. It’s then passed multiple times through a complex, energy-consuming neural network that progressively converts the noise to an image closer to the real images it has been trained on. The denoising step is repeated as many as a thousand times to arrive at a natural-looking image—taking up significant time and computing resources.
So, the team came up with an ambitious plan: to whittle the process down to one step. They were inspired by existing research on distillation methods in which researchers used pre-trained models to create pairs of before-and-after images—the noisy one at the beginning and the realistic one at the end. Then, with a dataset of pairs, researchers could train a smaller, nimbler neural network to create images more quickly by essentially shortcutting the iterative process. The early research was promising, but image quality tended to be garbled, distorted, or blurry.
At the time, Yin and Gharbi were also reading about 3D research that used a related approach, called score distillation sampling, to construct 3D models with supervision from a pre-trained image generative model. They decided to build on the existing research and find a new way to optimize the sampling process of those image generators.
“If you can create models with one one-thousandth of the steps required by the big models, that means you can start generating imagery in real time. And that opens up a very different way of interacting with image editing and creative workflows,” says Gharbi.
The possibilities of real-time, interactive image generation
With a much faster model for generating images, the team imagines the possibility of interacting in real-time with generative imaging tools. For example, a person could give text inputs or rough sketches for the basic outlines of an image, revising the inputs along the way, while the model simultaneously generates and updates a realistic version. Or users could get an instant, realistic preview of a complete scene from a coarse 3D rendering. The same method could improve generation for 2D images, 3D images, and even audio and video.
By using fewer steps, the technology also has the potential to speed up the entire AI generation pipeline while reducing costs and energy consumption.
“This project is such a good example of Adobe’s connection to the academic world, and the opportunities for interns at Adobe Research,” says Gharbi. Adobe Research’s internships “allow students to release work publicly so that others can build on it, and their projects can even have product impact. It’s a wonderful, mutually beneficial relationship.”
Wondering what else is happening inside Adobe Research? Check out our latest news here.