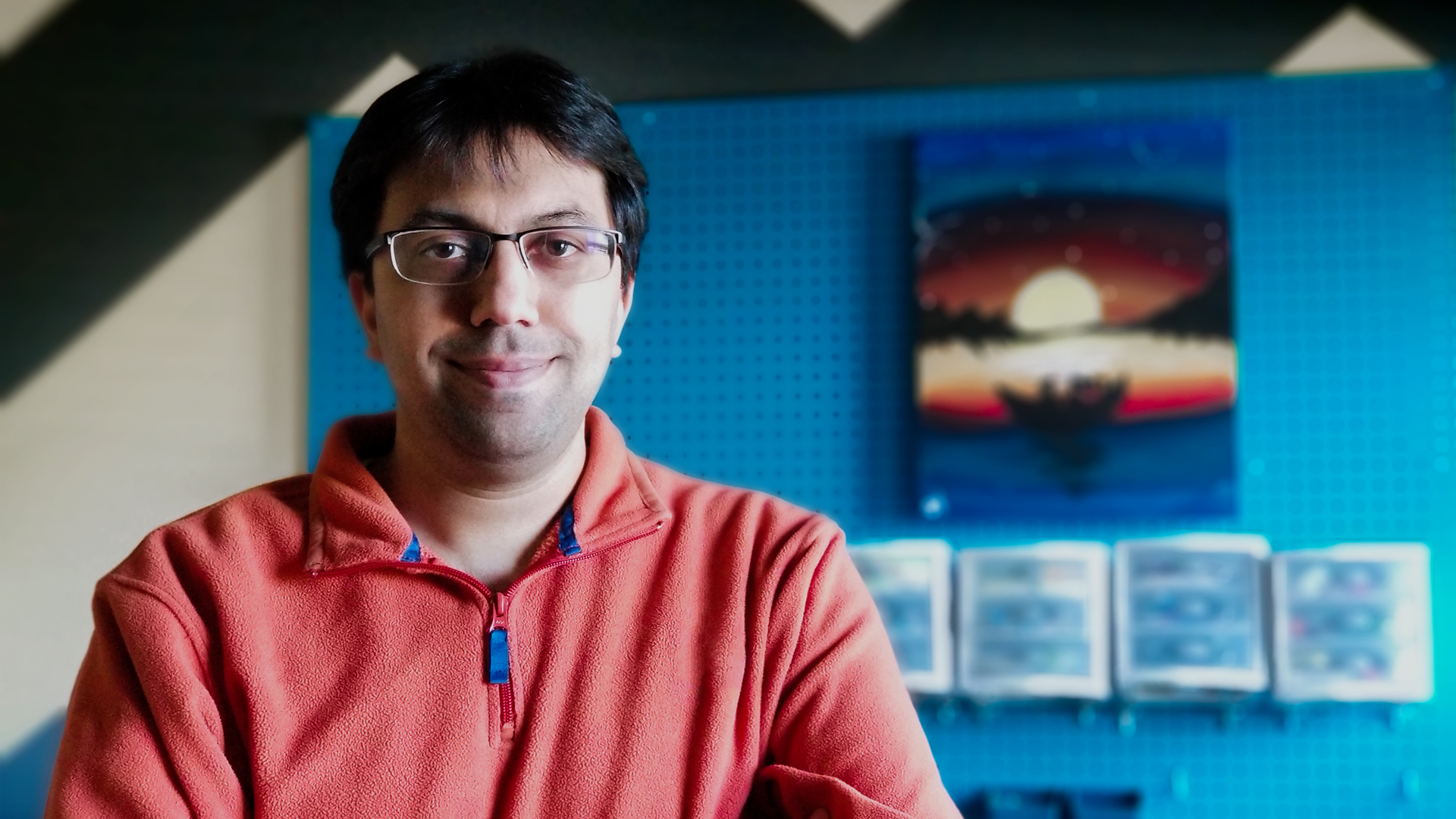
By Meredith Alexander Kunz, Adobe Research
Senior Research Scientist Rajiv Jain knows the pain of being surrounded by mountains of information, buried deep inside unstructured documents and media.
Before joining Adobe Research, Jain worked for the Department of Defense (DoD), first as an undergraduate intern, then as a network and forensic analyst. Finding relevant nuggets of intelligence amidst the noise proved a challenge, not just for Jain but for many colleagues. “It was painful for me to see analysts being overwhelmed when much of their daily work could be automated,” he recalls.
The stakes were high in an agency dependent on accurate information, so Jain began a brainstorm that would eventually lead him to a future at Adobe: Could computer vision and artificial intelligence help us understand what we need to know from a set of documents and media without having to examine them piece by piece?
To answer this question, Jain became a researcher. At DoD, he leveraged computer vision to design large-scale retrieval systems and build early cloud computing systems. His interests led him to pursue a PhD at the University of Maryland, College Park, where he dug deeper into document intelligence research. In 2018, after earning his PhD at the intersection of computer vision and document retrieval/analysis, and then consulting for DARPA on media forensics, Jain joined Adobe Research’s Maryland lab focused on document intelligence.
From analyzing national intelligence data to advancing the latest AI models, Jain has made headway into our complicated relationship with documents. We asked him a few questions to learn more.
How do you pick research problems to work on?
I’ve always been motivated by going after real-world problems. I am a very practical person, and I like to work on problems where I can actually see a use case. I have observed that new technologies do not always make their way from state-of-the-art research to the people who need them most. In my research work, I think: If I can at least solve one problem for one person, that’s good, and hopefully many people will benefit.
What are you working on now, and who are you trying to help?
My main focus now is bringing intelligence into contracts and the signing process. My wife is a contracting officer, and I can see that new technologies could help her and her colleagues.
For example, we have worked on an experimental tool that could take advantage of the structure of a document—its titles, headers, italics, and more. Our system learns from that structure, and it enables us to tailor our natural language models to a specific kind of document.
If you take a contract as an example, think about people’s needs. They want to know who else signed it, who promised to do what, the dollar amounts listed, and so on. Now if we apply this language modeling technology to contract specific data, it can make it much easier for people to find and extract what they care about.
What is language modeling, and how is it advancing your work?
Language modeling is about understanding the distribution of words in text. You see this when you use an online search engine—the system tries to predict the next words given what you’ve already typed.
There’s a recent breakthrough in the language community with machine learning: we’ve found that language models can teach themselves what is inside a sentence. This makes it much, much easier to understand a document. Once a model has been able to teach itself about language, it is much easier for the model to learn more specific things such as: what is a contract clause? What is a monetary value in a contract?
Are you working on ways to make documents more accessible?
Yes. Our team has an ongoing collaboration with the Trace Center at the University of Maryland (UMD), College Park, and we are exploring how to make accessibility easier for PDF documents. Our focus is on people who have low vision or are blind.
It’s a challenge. Our work with UMD is focused on taking advantage of our breakthroughs in understanding a PDF, so that publishers and authors can make them more accessible. We are exploring combining machine-learning/AI with a smarter user interface to improve the experience.
Has your work been incorporated into any Adobe products?
Some of my work was incorporated into Adobe Scan. We built a real-time machine learning boundary-detection model that could run on a mobile device. As you are scanning a document, this system automatically recognizes where the document is. It finds 4 dots to form boundaries around what’s to be scanned. Colleagues from Research involved included Curtis Wigington, Vlad Morariu, and Chris Tensmeyer.
Where do you see the future of document intelligence heading?
Recently the field has been driven by advances in deep learning. Research from computer vision has helped us understand the layout and structure of documents. And deep learning language models help us know what’s inside them. In the future, we’ll investigate how we can make it easier to train deep learning models and adapt them to new domains.
Right now, work in this field is also affected by COVID-19. At Adobe Research, we were expecting to do in-person, in-lab studies with users. We have had to figure out how to do this remotely and had to change our research questions in some cases. I think we’ve adapted well.
What’s it like to work at Adobe Research?
Adobe Research is an amazing place to work. First, I enjoy the opportunity to work with some of the most talented researchers in these fields, and the intersections among all of them. We have a lot of freedom in terms of research choices. Second, I love having the ability to take something from just an idea and get it all the way into a product, impacting millions of our customers: to make it easier for them to capture an image of a document, read better, or even understand a contract.