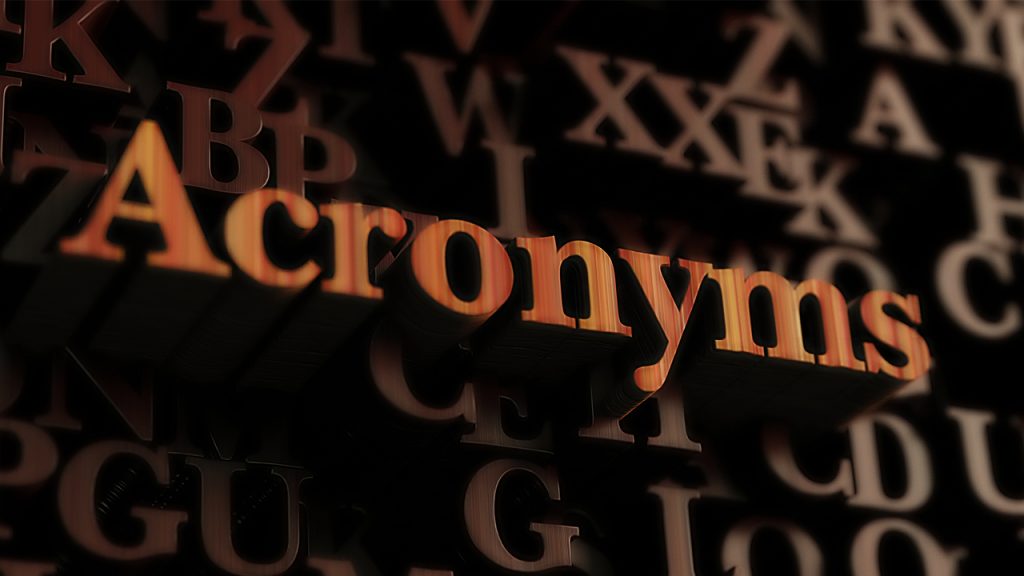
By Amir Pouran Ben Veyseh, Nicole Meister, Franck Dernoncourt, and Walter Chang, Adobe Research
An acronym is the shorter form of a longer phrase. For example, in the sentence “Adobe invented the Portable Document Format (PDF) to present and exchange documents reliably,” the term “PDF” is an acronym, and “Portable Document Format” is its long form.
On the one hand, acronyms facilitate communication and reading by avoiding the use of long phrases. However, on the other hand, they might decrease the readability of a text if the reader is not familiar with the acronym. As such, it is important to provide the long form of acronyms to the reader for a better understanding of the text.
In an Adobe Research paper presented at EACL 2021, which won the best paper award in the demonstration track, we introduce a new system to find the long form of an acronym present in a text. Our system is able to perform two tasks, both referred to as acronym expansion:
- It extracts the long forms of the acronyms from the text, in case the long form is present in the text.
- It predicts the long forms of the acronyms, in case the long forms are not present in the text.
This is valuable to people reading a wide range of texts that include complex acronyms, such as scientific papers.
Our system has three major advantages compared to existing solutions.
First, it covers more types of acronyms. While previous methods are restricted to certain domains, our system supports a variety of domains from biomedical to chemistry and general domain. This wide coverage comes from the large corpora from several domains employed to build the system (some contain more than 3 billion sentences). Currently, our system supports more than 426,000 acronyms with more than 3 million long forms.
Second, our system is able to expand both locally-defined acronyms and acronyms with non-local definitions. Some of the acronyms might be defined in the input document, but for some reasons (for instance, when the acronym is frequently used in the domain) the writer might skip providing the long form for some of the acronyms. Since an acronym typically has multiple possible long forms, an acronym expansion system should be able to also perform acronym disambiguation to find the correct long form of an acronym from a large dictionary, in case the acronym’s long form is present in the input document. The acronym disambiguation component of our system is trained on a massive dataset with more than 46 million training samples.
Third, our system is thoroughly evaluated on two benchmark datasets. This analysis showed that our system has stronger performance compared to the existing models for acronym expansion.
This work is part of our broader initiative to find the definitions of any elements in a text to make reading more efficient: complex words, acronyms, mathematical symbols, and more.
References for our work on acronyms:
MadDog: A Web-based System for Acronym Identification and Disambiguation. EACL 2021 System Demonstrations. Amir Pouran Ben Veyseh, Franck Dernoncourt, Walter Chang, Thien Huu Nguyen. Best Paper Award.
Acronym Identification and Disambiguation shared tasks for Scientific Document Understanding. AAAI 2021 Workshop on Scientific Document Understanding. Amir Pouran Ben Veyseh, Franck Dernoncourt, Thien Huu Nguyen, Walter Chang, Leo Anthony Celi.
What Does This Acronym Mean? Introducing a New Dataset for Acronym Identification and Disambiguation. COLING 2020. Amir Pouran Ben Veyseh, Franck Dernoncourt, Quan Hung Tran, Thien Huu Nguyen.
References for our work on extracting definitions for any term in a text (not specific to acronyms):
A Joint Model for Definition Extraction with Syntactic Connection and Semantic Consistency. AAAI 2020. Amir Pouran Ben Veyseh, Franck Dernoncourt, Dejing Dou, Thien Huu Nguyen.
SemEval-2020 Task 6: Definition Extraction from Free Text with the DEFT Corpus. SemEval-2020. Sasha Spala, Nicholas Miller, Franck Dernoncourt, Carl Dockhorn.
DEFT: A corpus for definition extraction in free- and semi-structured text. ACL LAW-XIII-2019. Sasha Spala, Nicholas A Miller, Yiming Yang, Franck Dernoncourt, Carl Dockhorn.