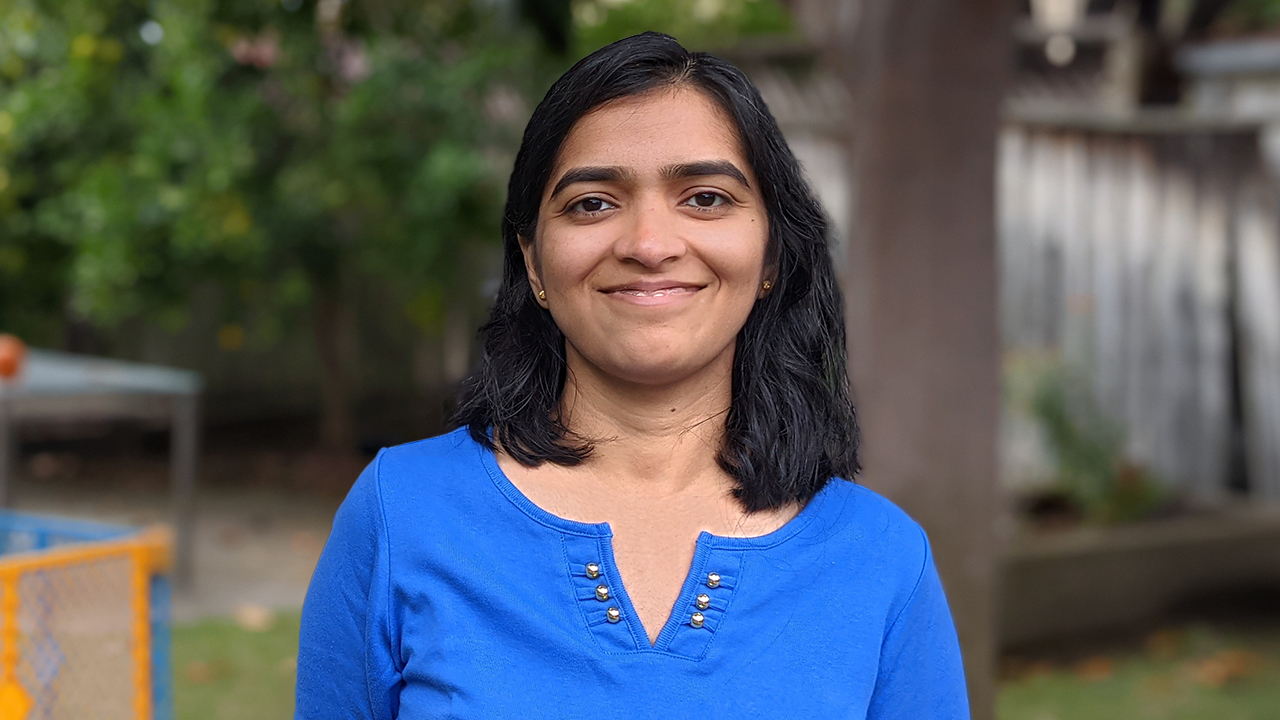
Research Scientist Kanak Mahadik uses machine learning so that large-scale systems—like Adobe’s cloud-based services—can use resources better. With greater efficiency, Adobe can improve the experience for customers, lower operating costs, and use less energy, reducing our impact on the environment.
Mahadik talked to us about how machine learning can improve performance, what she’s learned about the interactions between people and AI, and why Adobe Research is a great place to tackle big questions.
What are you working on at Adobe Research right now?
I’m developing intelligent and efficient cloud resource allocation and forecasting algorithms using machine learning techniques, and communication-efficient distributed systems for online recommendation algorithms. Right now, my work is helping the cloud team at Adobe to deliver higher performance for our customers.
What kinds of problems are you solving with your work?
Traditionally, big systems have a set of configuration parameters that can be tuned for optimal performance, but managing those parameters takes a lot of manual effort and skill. And at the scale systems operate today, it’s very difficult to do the performance tuning. It’s time consuming, and not very effective.
To help solve this problem, we’re looking at the data collected for large systems. In the case of cloud applications, there’s data around the CPU, memory usage, and logging of different applications. We can use all of this data to apply new machine learning techniques.
This work is helping the Adobe cloud infrastructure team—they’re responsible for deploying and hosting services across multiple clouds. We have already had an impact of saving several million dollars for the Adobe bottom line, and we’ve made it possible to enable new functionality and features for Adobe customers—which is quite delightful for us.
What have you learned about how people want to interact with machine learning technology?
We were interested in understanding the level of detail and analysis that users want to see before they accept a machine learning system’s suggestion, especially when they are used to doing the work manually. So, what needs to be in the interface to convince users to accept our recommendations?
We discovered that before applying changes, users wanted to see what the results will look like. And they want to see a few scenarios that aren’t necessarily recommended—which surprised us. The recurring theme was really that we needed to allow the human to be in the loop. The humans looked at the ML recommendations and applied their own knowledge to make decisions.
How did your interests bring you to Adobe Research?
I did my PhD at Purdue University, and I was looking for a balance between academic research—attending conferences and reading and writing papers—and doing applied research that solves practical problems and actually runs in production systems.
I knew I wanted to work in industry because there are unique and challenging problems that only occur when products and systems run at a large scale. These challenges are completely absent when a system is run in a prototype mode, for example at a university. But they appear at truly large scales, such as Adobe production systems. I found that Adobe Research had the right balance between being able to work on real-world problems and being able to contribute to the academic field.
Another part of Adobe Research that I love is the internship program, which gives us the opportunity to mentor bright students at varying levels in their PhD programs. It’s a learning experience every time, so it’s something I look forward to every year.
I was also drawn to Adobe Research because of the diversity of research areas, and the opportunity to think at the intersection of different areas and come up with innovative solutions. It’s genuinely fun to discuss problems with colleagues who are working in different fields—it’s always an open conversation, and there are a lot of interesting solutions that come about.
Interested in working with our team of research scientists and engineers? Learn more about careers with Adobe Research!