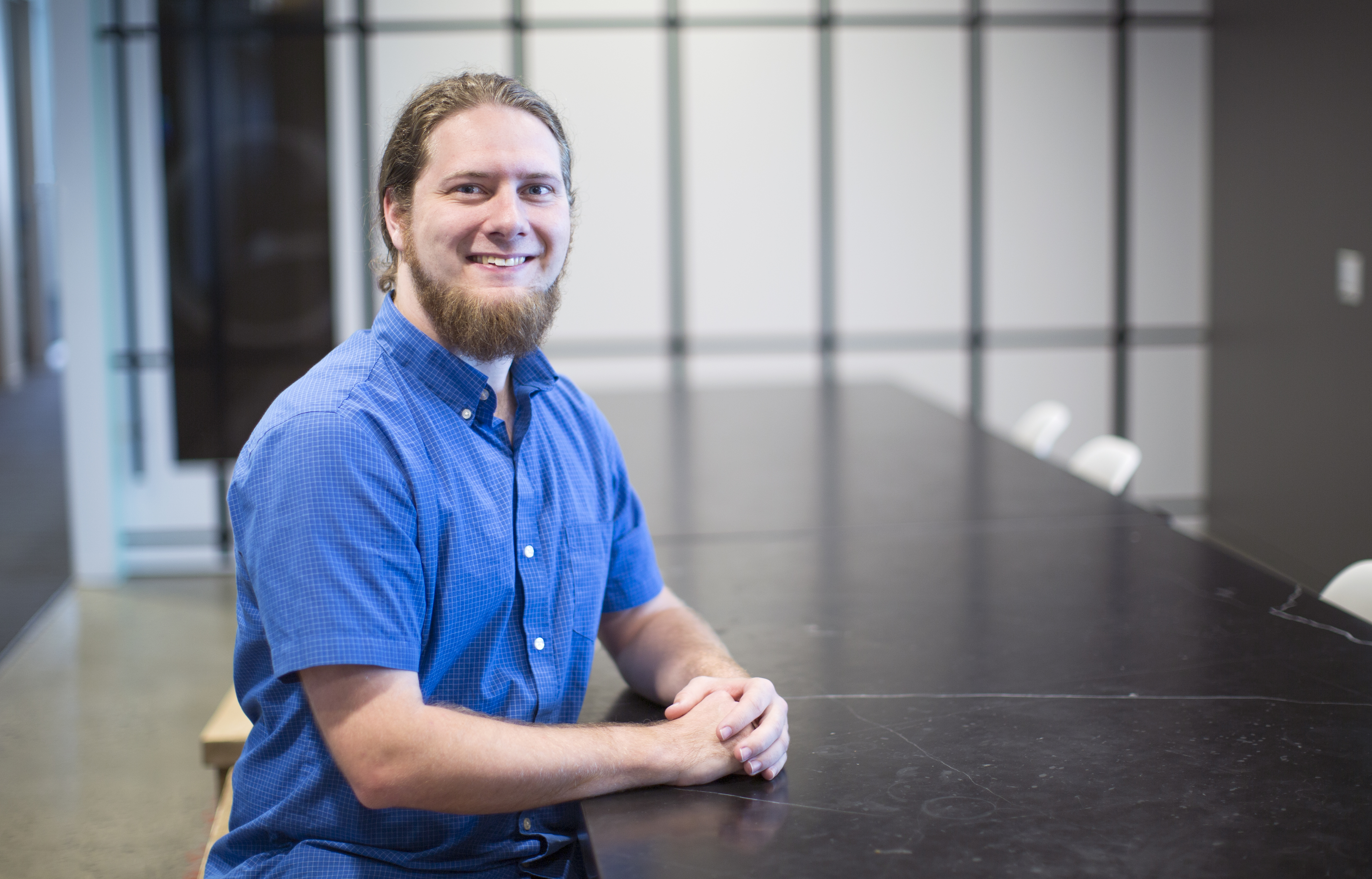
By Meredith Alexander Kunz, Adobe Research
Yannick Hold-Geoffroy, a research engineer, never thought his PhD dissertation would win an award. “I sincerely was not expecting it. I was just happy I’d finished my PhD, and super happy I ended up working at Adobe Research,” he says.
This spring, the Quebec City native discovered he’d been selected as one of two winners of a prestigious national prize from the Canadian Image Processing and Pattern Recognition Society (CIPPRS). The annual CIPPRS Doctoral Dissertation Award from this group covers a wide range of topics included in the group’s Conference on Computer and Robot Vision. That incorporates computer vision, robot vision, robotics, medical imaging, image processing, and pattern recognition. Recipients must have completed their dissertation at a Canadian institution.
Hold-Geoffroy traveled to receive the award in late May. It honored his thesis, entitled “Learning Geometric and Lighting Priors from Natural Images” (the entire dissertation can be found here). He earned his doctorate working with Jean-François Lalonde and Paulo Gotardo at Laval University.
He describes his dissertation as a quest to answer this fundamental question in computer vision: “How do I use deep learning to find out, among a large family of solutions, which are the most plausible ones to explain why an image looks the way it does?”
For example, looking at any natural image, you will see geometries and shadows. As humans, using our visual perception, we can reason about an image: noticing a pedestrian, for example, we may see that the person is standing up, walking, and casting a shadow. But computers do not understand imagery at that level—yet.
“I tried to set some concrete tasks for the system, such as finding the position of the sun and the amount of clouds. From there, I tried to get the AI to become better at understanding those elements, and in general, what it is seeing in the image,” Hold-Geoffroy explains.
It’s a very ambitious goal. Ultimately, Hold-Geoffroy says that this kind of effort will help computers understand more of the world in a more human-like way.
In the meantime, his work is also exploring how the computer understands at a more basic level. He is probing the so-called “black box” inside machine learning—the interior workings of the system not always visible to humans—to discover how neural networks conclude what is in an image.
“In part of my dissertation, I looked at what regions of an image would most change the machine’s decision,” he explains. In one case, Hold-Geoffroy examined a computer that was engaged in a geometry task. “I found out the machine was looking at vanishing lines, for example, even though we never trained it to look at that. That’s what it was doing under the hood.”
Today, Hold-Geoffroy’s curiosity is propelling more work along these lines at Adobe Research. Some of his discoveries are bolstering 3D image compositing for Adobe’s software, and he has contributed two shipped features in Adobe Dimension.
“This work could also apply to augmented reality,” Hold-Geoffroy adds. “If we want the things we add to augment the world to look realistic, we need an understanding of what’s happening in the scene, especially lighting. If that’s not done well, it won’t look right.”
Photo by Claire (Qin) Li