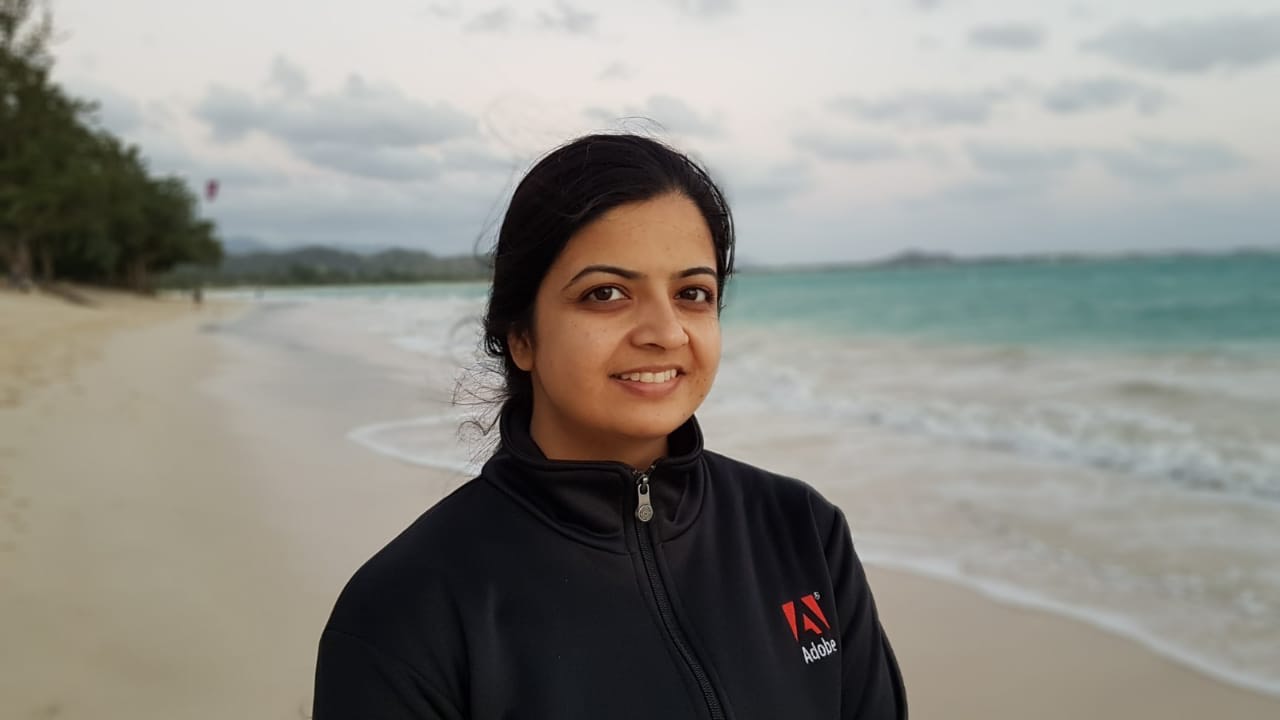
Ever been frustrated by language online? These days, it’s a near universal experience. Luckily Senior Research Scientist Niyati Chhaya of Adobe Research is here to help us understand how emotions are expressed and felt through language in emails, websites, and social media. Her work empowers enterprises to do a better job connecting with consumers in positive ways.
Chhaya studies the many kinds of text that we read and write on our computers. Her work spans several cutting-edge fields: natural language processing, machine learning, “affective” computing, and psycholinguistics.
At Adobe Research, Chhaya has contributed technologies to Adobe Experience Manager, Adobe Campaign, and Adobe Social. She’s also active in the academic community. Her recent research papers include Frustrated, Polite or Formal: Quantifying Feelings and Tone in Emails and Diachronic Degradation of Language Models. The Bangalore-based researcher has also mentored more than 20 interns.
What does your research focus on?
I work on how to quantify and measure the experience of emotions, or any human reactions, to content on our computers. This field is called affective computing and affective content analysis. Predominantly, I work on text.
In the digital marketing space, I focus on this question: how do you create content that caters to various different user groups? For example, if I want to create something for a teenager, I’d use very informal language. For managers, I’d keep it polite and formal.
In the process, we develop tools that make predictions and do scoring for what kind of content does well. A key question drives my work: Is this going to frustrate someone or not?
What is psycholinguistics?
With this approach, you are studying human psychological traits in language. For example: “Children are playing in the park. It’s sunny outside.” That kind of text would leave the reader in a pleasant psychological state. But what about, “Children are playing in the park. We heard a gunshot nearby.” That will generate anxiety. The language is related to the context. Through psycholinguistics, we are trying to quantify the psychological state both of the author and the consumer using their language footprint.
What kind of data do you use to explore these questions?
It’s a combination of two kinds of data. One is enterprise content—website content, marketing emails—and from that, I can get information about how it’s consumed through analytics. For example I can learn where the audience is coming from, or how many clicks are made on content on a given page. That way we get a measurement of whether that content worked or not.
The second kind of data is user generated content, which is predominantly social media data. That helps us understand how users write and how they react through content. If we want to build a model of teenagers who will like specific words vs. soccer moms—that model comes from user generated content. The models are then mapped to enterprise content, and we can see where we might change the wording.
How has your work made an impact on Adobe products?
Brand-specific user scoring and expert scoring features were added to Adobe Experience Manager over two years ago. Here’s how it works. Let’s say you have brand-based online communities or forums. People might want to reach out to specific users known to give good answers about a specific topic. For example, in a forum from a car company, participants might have questions about a certain model of car. User scores can help associate that question with an expert who knows about that model. Larger companies use this for consumer care.
I also worked on the prediction of email subject lines. Would you open an email or not, based on the subject? That was also included in a product, Adobe Campaign, a couple of years ago. Another technology I helped develop with Senior Research Scientist Balaji Vasan Srinivasan is called Smart Layouts. It automates layout creation for marketing materials. It was released in beta last fall in Adobe Experience Manager.
What role does machine learning play in your work?
Machine learning plays a significant role. We have no general definition to say a certain piece of content will generate or show happiness or sadness. To find this out, we rely on human-tagged data. Then you need to develop models computationally that associate a piece of text with a given affective, or emotional, trait. Deep learning, when we have enough data, is the go-to place to do this.
For example, machine learning helps us find frustration in texts we read. But there’s more: to remove the reader’s or the author’s frustration, I need to go back and look at how and what the model learned in more detail. What causes frustration? Is it longer sentences? Certain words? So, it’s not just using machine learning out of the box—it’s trying to do an analysis to see what the network learned. We’re aiming towards more interpretability in the machine learning space.
Based on an interview with Meredith Alexander Kunz